
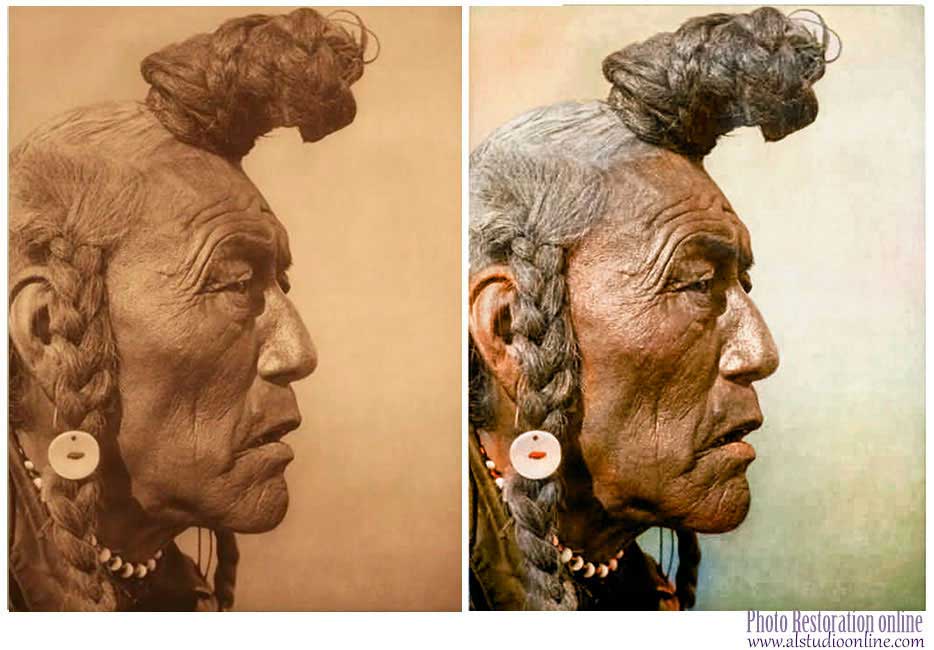
In a full color image, each pixel will have three numbers, one for H, S, and V. No, and to better understand this, it'd be worth learning about the HSV color model (hue, saturation, value.) Isn't it true that even black and white photos contain colour information Results of the first-few iterations will be terrible as the network weights are initialised with random noise, but after a few epochs of 'back-propagation' where neuron-weights are corrected and adjusted to minimise a loss function, colourisation quality improves.ĮDIT: changed 'image quality' to 'colourisation quality', I have a more layman-friendly explanation below. To do this the authors converted the millions of images from the ImageNet dataset into grayscale (recall that this can easily be done by merging all 3 colour channels), and having the network predict the original colours of the image in the HSV colourspace. The architecture was 'trained' to predict the colours of an image given some grayscale input.
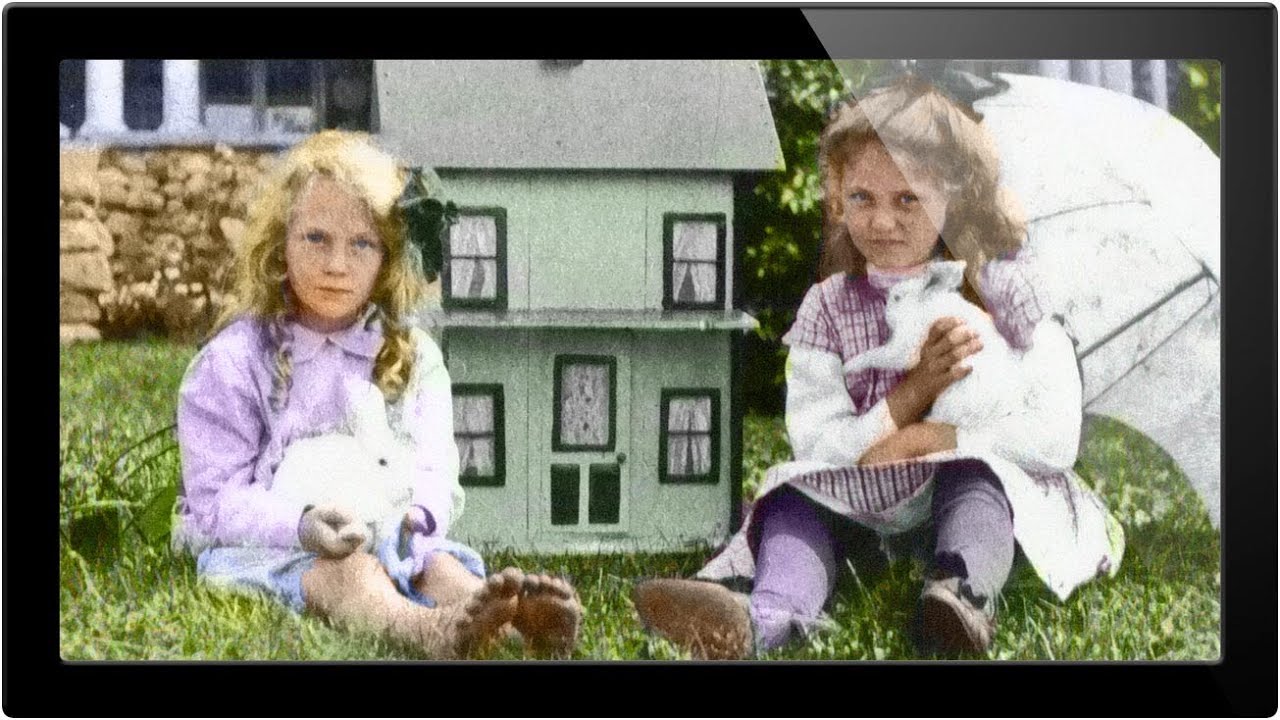
This architecture utilises a convolutional neural network (CNN, the Stanford course CS231n gives an excellent rundown) which gained popularity in 2012 when it revolutionised image classification on the ImageNet challenge (ImageNet Classification with Deep Convolutional Neural Networks, 2012).
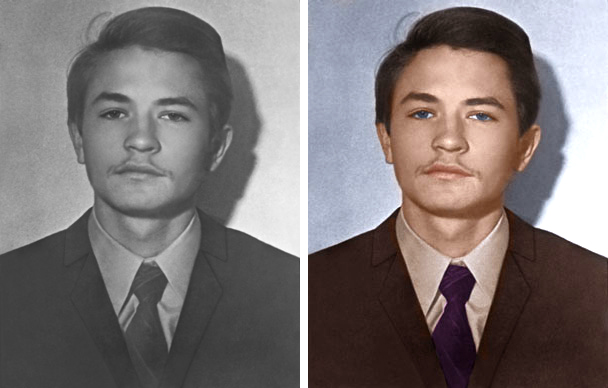.jpg)
A popular implementation is by Zhang (Colorful Image Colorization, 2016), powering colorizebot (/user/pm_me_your_bw_pics). However, with the hype surrounding deep-learning (DL) it is sinful to not mention how DL approaches colourisation.
COLORIZE OLD PHOTO PATCH
The latter is a collection of coloured 'reference' image whose colours are automatically retrieved by an algorithm based on the texture of the greyscale patch to colourise. Automated Colorization of Grayscale Images Using Texture Descriptors and a Modified Fuzzy C-Means Clustering, 2011). How traditional computer-science methods can help is with edge-detection algorithms that can define object borders (Canny, Sobel, etc), or information-retrieval approaches that attempt to colourise objects based on a 'texture bank' (e.g.
COLORIZE OLD PHOTO MANUAL
In its early stages, colourisation required a lot of manual work both with selecting the colours and identifying object boundaries.
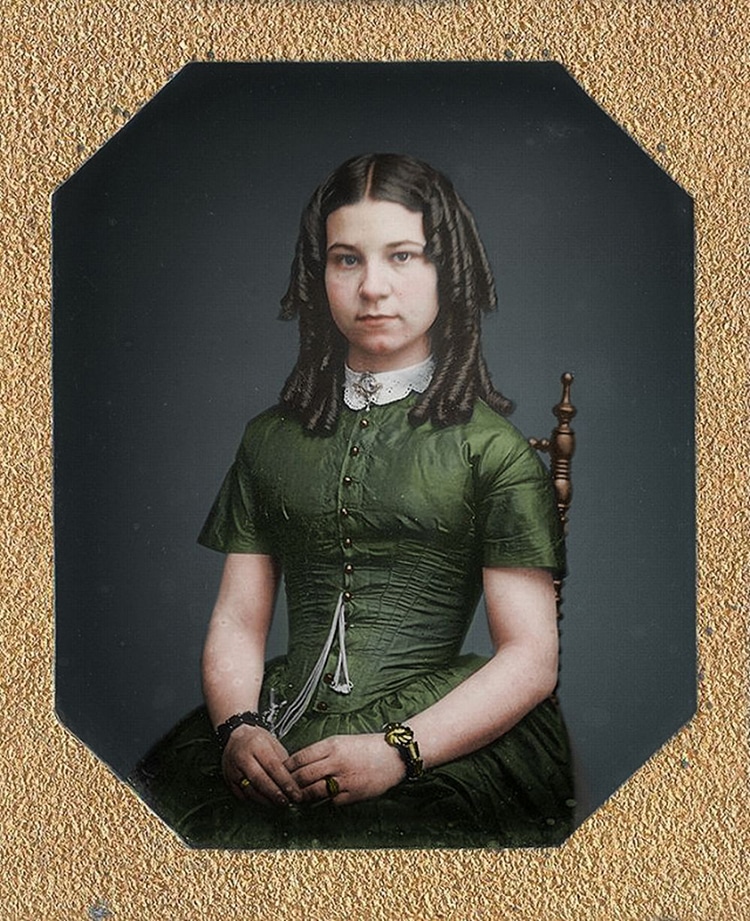
With the introduction out of the way let us now discuss traditional colouring methodologies, skimping over non-CS related detail such as colour selection. This can be simulated in an RGB colour image by setting all 3 channels to the same value. In contrast, grayscale images - what you call black-and-white images - represent the image with only one colour channel. These colours correspond roughly to the colour-sensitive photoreceptors in our eyes, hence why we have RGB screens. The conventional and most-popular form of representing coloured images is to separate the image in to three colour channels: RED, GREEN, and BLUE (RGB). Let's start with describing how colours are represented in an image, and what makes an image 'black-and-white'. Seeing that as /u/mfukar has removed a lot of comments, mentioning manual reconstruction or photo-editing I will refrain from discussing colourisation from that angle - however those methods are still very much applicable (computer-assisted manual colourisation). I do work in computer vision with applications in graphics.
